二进制逻辑回归
二项式逻辑回归详细操作教程
逻辑回归的最简单形式是二进制或二项式逻辑回归,其中目标或因变量只能具有2种可能的类型(1或0)。它使我们能够对多个预测变量与二进制/二项式目标之间的关系进行建模变量。在逻辑回归的情况下,线性函数基本上用作以下关系的另一个函数的输入,例如an-
$$ h _ {\ emptyset}(x)= g(\ emptyset ^ {T} x)其中0 \ leq h _ {\ emptyset} \ leq1 $$
在这里,𝑔是逻辑或S型函数,可以给出如下-
$$ g(z)= \ frac {1} {1 + e ^ {-z}}其中\:z = \:\ emptyset ^ {T} x $$
可以通过下图来表示S形曲线。我们可以看到y轴的值介于0和1之间,并且与该轴的交点为0.5。
这些类别可以分为正面或负面。如果输出介于0和1之间,则输出属于正类概率。对于我们的实现,我们将假设函数的输出解释为大于或等于0.5时为正,否则为负。
我们还需要定义一个损失函数,以使用权重来衡量算法的性能,以theta表示,如下所示-
$$ h \:= \:g(X \ emptyset)$$
$$ J(\ emptyset)\:= \:\ frac {1} {m}。(-y ^ {T} log(h)\:-\ :( 1-y)^ {T} \ :log(1-h)$$
现在,在定义损失函数之后,我们的主要目标是使损失函数最小化。可以借助调整权重来完成,这意味着可以增加或减少权重。借助损失每个权重的损失函数的导数,我们将能够知道哪些参数应具有较高的权重,哪些参数应具有较小的权重。
以下梯度下降方程告诉我们,如果我们修改参数,损耗将如何变化-
$$ \ frac {\ partial J(\ emptyset)} {\ partial \ emptyset_ {j}} \:= \:\ frac {1} {m} \:X ^ {T} \ :( g( X \ emptyset)\:-y)$$
Python的实现
现在,我们将在Python中实现上述二项逻辑回归的概念。为此,我们使用了一个名为" iris"的多元花卉数据集,该数据集具有3类,每类50个实例,但是我们将使用前两个要素列。每个班级代表一种鸢尾花。
首先,我们需要按如下所示导入必要的库-
# Filename : example.py
# Copyright : 2020 By Lidihuo
# Author by : www.lidihuo.com
# Date : 2020-08-27
import numpy as np
import matplotlib.pyplot as plt
import seaborn as sns
from sklearn import datasets
接下来,按如下所示加载虹膜数据集-
# Filename : example.py
# Copyright : 2020 By Lidihuo
# Author by : www.lidihuo.com
# Date : 2020-08-27
iris = datasets.load_iris()
X = iris.data[:, :2]
y = (iris.target != 0) * 1
我们可以如下绘制训练数据-
# Filename : example.py
# Copyright : 2020 By Lidihuo
# Author by : www.lidihuo.com
# Date : 2020-08-27
plt.figure(figsize=(6, 6))
plt.scatter(X[y == 0][:, 0], X[y == 0][:, 1], color='g', label='0')
plt.scatter(X[y == 1][:, 0], X[y == 1][:, 1], color='y', label='1')
plt.legend();
接下来,我们将定义S型函数,损失函数和梯度递减,如下所示-
# Filename : example.py
# Copyright : 2020 By Lidihuo
# Author by : www.lidihuo.com
# Date : 2020-08-27
class LogisticRegression:
def __init__(self, lr = 0.01, num_iter = 100000, fit_intercept = True, verbose = False):
self.lr = lr
self.num_iter = num_iter
self.fit_intercept = fit_intercept
self.verbose = verbose
def __add_intercept(self, X):
intercept = np.ones((X.shape[0], 1))
return np.concatenate((intercept, X), axis=1)
def __sigmoid(self, z):
return 1 / (1 + np.exp(-z))
def __loss(self, h, y):
return (-y * np.log(h) - (1 - y) * np.log(1 - h)).mean()
def fit(self, X, y):
if self.fit_intercept:
X = self.__add_intercept(X)
现在,按如下所示初始化权重-
# Filename : example.py
# Copyright : 2020 By Lidihuo
# Author by : www.lidihuo.com
# Date : 2020-08-27
self.theta = np.zeros(X.shape[1])
for i in range(self.num_iter):
z = np.dot(X, self.theta)
h = self.__sigmoid(z)
gradient = np.dot(X.T, (h - y)) / y.size
self.theta -= self.lr * gradient
z = np.dot(X, self.theta)
h = self.__sigmoid(z)
loss = self.__loss(h, y)
if(self.verbose ==True and i % 10000 == 0):
print(f'loss: {loss} \t')
借助以下脚本,我们可以预测输出概率-
# Filename : example.py
# Copyright : 2020 By Lidihuo
# Author by : www.lidihuo.com
# Date : 2020-08-27
def predict_prob(self, X):
if self.fit_intercept:
X = self.__add_intercept(X)
return self.__sigmoid(np.dot(X, self.theta))
def predict(self, X):
return self.predict_prob(X).round()
接下来,我们可以评估模型并将其绘制如下-
# Filename : example.py
# Copyright : 2020 By Lidihuo
# Author by : www.lidihuo.com
# Date : 2020-08-27
model = LogisticRegression(lr = 0.1, num_iter = 300000)
preds = model.predict(X)
(preds == y).mean()
plt.figure(figsize = (10, 6))
plt.scatter(X[y == 0][:, 0], X[y == 0][:, 1], color = 'g', label = '0')
plt.scatter(X[y == 1][:, 0], X[y == 1][:, 1], color = 'y', label = '1')
plt.legend()
x1_min, x1_max = X[:,0].min(), X[:,0].max(),
x2_min, x2_max = X[:,1].min(), X[:,1].max(),
xx1, xx2 = np.meshgrid(np.linspace(x1_min, x1_max), np.linspace(x2_min, x2_max))
grid = np.c_[xx1.ravel(), xx2.ravel()]
probs = model.predict_prob(grid).reshape(xx1.shape)
plt.contour(xx1, xx2, probs, [0.5], linewidths=1, colors='red');
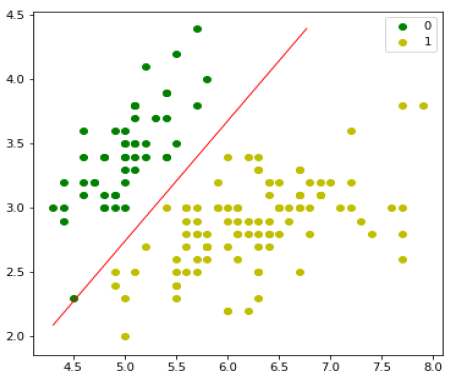